In this article
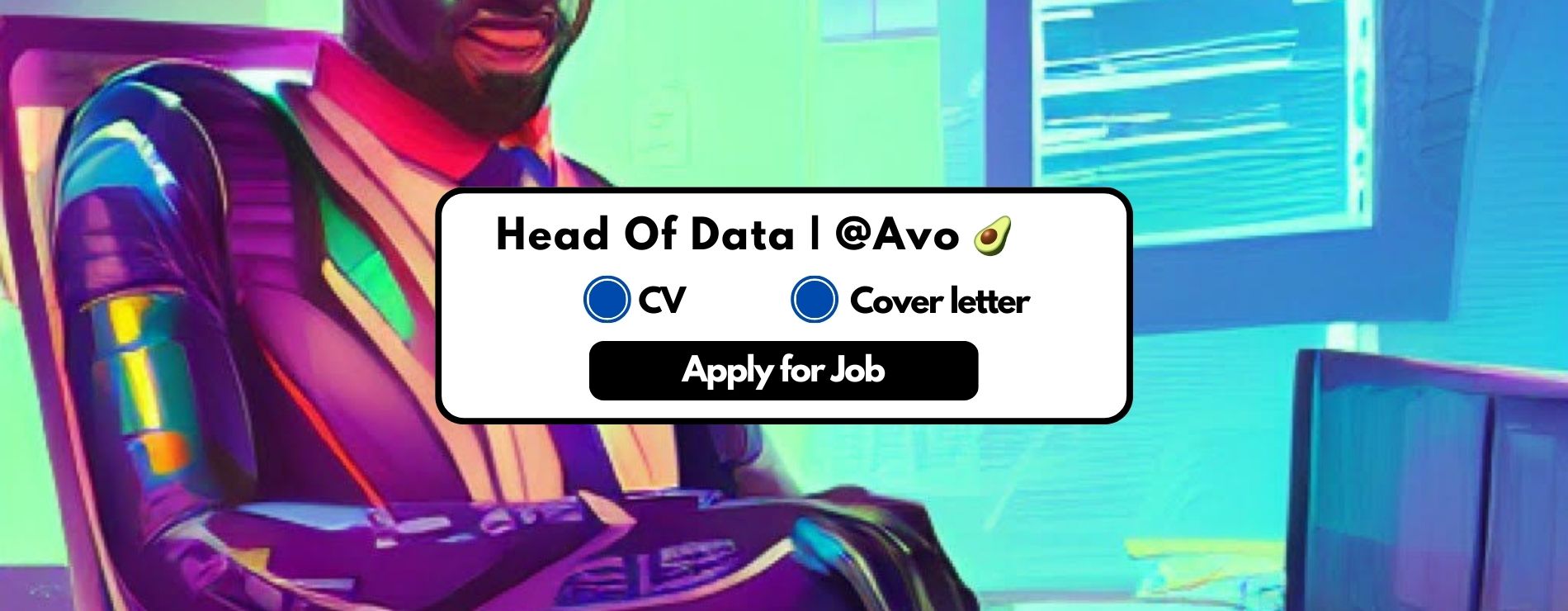
6 Best Data Jobs in 2023 and How To Land Them
Top 6 Data Jobs 2023 · 1. Data analyst · 2. Data product manager · 3. Data scientist · 4. Data engineer · 5. Machine learning engineer · 6. Analytics engineer
Despite news of hiring freezes and layoffs becoming the order of the day, data skills are still in high demand. Research by the US Bureau of Labor Statistics (BLS) states that data-related occupations are expected to grow by more than 31 percent by 2031. Another study from Glassdoor reports that many of the most in-demand skills in 2022 are related to data.
Whether you have been affected by layoffs, are looking to transition to the data field, or are seeking a new role, a career in the data sector offers lucrative and rewarding benefits. Here are the five best data jobs to consider for your next role.
DISCLAIMER: Ownership and responsibility around data is a topic for hot debate, and the cross over between the roles that we have outlined below can often blur. That said, speaking with our customers, we’ve learned that companies often struggle to find people with data skills and the demands for shipping quality data products fast only grows with the business, so our recommendation would be to send that job application, even if you don’t fit the role exactly as it was typed up by HR 😉. We can confidently say that even in this economic downturn, you’re needed now more than ever!
1. Data analyst
Data analysts create reports, dashboards, and other visualizations of data to provide insights in support of decision-making efforts and to help solve specific problems. For example, analysts help answer questions such as, “What is our highest-performing product?” and, “How many signups did the last marketing campaign deliver?”
What are data analysts’ responsibilities?
On a day-to-day basis, data analysts:
- Use statistical tools to identify and analyze trends and patterns in data sets
- Design and prepare reports and dashboards for a diverse audience to communicate trends, patterns, and predictions in data sets
- Automate data collection and reporting processes
- Collaborate with both technical and non-technical users to understand their analytical needs and effectively use data to identify opportunities for process improvements
- Define new data collection and analysis processes
What skills do data analysts need?
Data analysts are expected to be proficient in a variety of technical and soft skills, including:
- Data querying: Data analysts are often tasked with writing queries to retrieve and analyze data from diverse sources (databases, spreadsheets). Therefore, knowledge of querying with SQL and Excel is an essential skill for data analysts.
- Statistics and programming: A combination of coding in programming languages like Python and R, with some theoretical background in statistics, allows data analysts to analyze large sets of data and perform computations.
- Data visualization: Learning to present your findings clearly and compellingly is necessary for becoming a successful data analyst. Power BI and Tableau are some tools data analysts use to deliver their data.
- Verbal and written communication skills: A significant part of a data analyst’s skill is clearly and concisely presenting findings to decision-makers and other company stakeholders to help support data-driven decision-making.
Here are some good resources if you want sharpen your data analytic skills.
- data analyst training course by Google (Free).
- simulator for data-driven product management by GoPractice (paid)
- Short data courses for data-driven marketers by Vexpower (free/paid)
2. Data product manager
As companies mature and begin to treat data as a product, the data product manager is responsible for data democratization and increasing the time to value for the data itself. They design, build and manage the cross-functional development of a data platform, facilitating the access and use of data, or a suite of specific data tools and processes, to serve multiple customers.
What are Data product managers’ responsibilities?
- Support with data accessibility, quality, and security when delivering analytics products.
- Designing process, stakeholder alignment, and advising on data product development.
- Collaborating with engineering, product, and other business teams to uncover new data product opportunities, and guide the business through solution ideation and the technology landscape.
- Improve data quality attributes so that the business can act on reliable insights and provide great customer experiences.
What skills do Data product managers’ need?
- Technical project management: Data product innovation can often suffer scope creep. Therefore experience in managing innovation in technical environments is helpful.
- Interpersonal skills: It’s common for the lines of responsibility to be blurry creating frustrations that need to be navigated. Being able to diffuse conflicts and facilitate productive discourse amongst different stakeholders is essential. You’ll likely need to roll out changes to a way of working, so knowing when to be firm or when to take a soft approach will help you win over hearts and minds.
- Organization & collaboration: The demands on data only grow with an organization, so knowing how to prioritize and schedule projects with complex dependencies will help you set good expectations and flag the need for more resources when time constraints are critical.
- Data design: Garbage in garbage out they say, so knowing what good looks like will help you roll out initiatives to improve overall confidence in data.
What is the preferred tool of the Data product manager?
Avo is fast becoming the tool of choice for the data product manager for data quality in product analytics. Avo helps teams like Ikea, Wolt, Fender, Sixt and Condé Nast to plan, implement and verify analytics, so they don't fly blind and fail to build great user experiences.
Why do Data product managers love Avo
Data design guide rails: Avo provides a visual interface for designing new event tracking, with features and guardrails to both reduce the complexity of designing high quality data, and speed up the entire process. This helps data product managers avoid becoming the bottleneck. Feature highlights:
- Tracking plan branched workflows: lets multiple teams work on tracking changes safely.
- Tracking plan audit rule: catches poor data design before release.
- Event screenshots help teams understand where an event is triggered.
- Event property groups saves you time when adding multiple event properties with a single click.
- Publishing automatically syncs your schema (including event descriptions) across your stack.
Tracking observability: Avo Inspector audits the tracking implementation against the planned schemas while the data product manager sleeps, and flags data bugs in the interface, with configurable alerts to Slack or into any tool that can receive from a webhook. Data product manager will use Inspector to reduce data downtime of mission critical events, as well as reduce data debt overtime. Feature highlights:
- Inspector: catches data bugs while you sleep.
- Slack alerts alerts you when tracking breaks.
Developer Collaboration: Avo lets you auto-generate a markdown implementation guide for a developer, so that you can copy paste the instructions for the needed tracking changes. With features that let you monitor the progress of implementation or align different stakeholders around important metrics or needed schema design nuances. Feature highlights:
- Implementation instructions: lets you copy paste dev tech specs.
3. Data scientist
A data scientist applies machine learning and algorithmic approaches to large data sets by creating models that predict customer behavior, provide recommendations, and spot patterns in data sets. As data volumes increase and more companies are better equipped to personalize their customer experiences, and find cost saving opportunities, the data science field is becoming increasingly popular.
What are data scientists’ responsibilities?
Although data scientists and data analysts’ duties are frequently confused, they have very different responsibilities. Data analysts examine existing data to identify trends and draw conclusions, while data scientists often deal with the unknown, using more advanced data techniques to make predictions for the future.
Some of the responsibilities of data scientists include:
- Applying predictive modeling and machine learning techniques to mine big datasets
- Designing workflows, providing recommendations, and driving success on end-to-end analytics initiatives
- Proactively performing data exploration on large datasets to discover future opportunities
- Collaborating with engineering and product development teams to uncover new opportunities, analyze experiments, and deliver solutions
What skills do data scientists need?
The data scientist role is quite technical, and while many companies require an advanced degree in statistics, mathematics, or a related quantitative field, we’ve seen an increasing number of new data scientists get hired right out of 3-month data science bootcamps. General skills needed in this role include:
- Probability and statistics: Making inferences, estimating, and predicting form a vital part of the data science role, so a good knowledge base in statistics and probability will help you succeed in this role.
- Data wrangling: Data scientists might often have to transform and map their raw data from one form to another to prepare it for modeling.
- Machine learning: Data scientists often incorporate machine learning techniques to gather more information faster or assist with trend analysis.
- Programming languages: Programming skills help combine all the fundamental skills needed to create machine learning algorithms and organize unstructured datasets. While there is no specific rule about selecting programming languages, Python and R are the most favored.
- Data visualization and communication: Data scientists often have to present their findings to other stakeholders.
Several universities and online learning providers offer a Master of Data Science degree or shorter courses with coaching, which can give you the necessary skills to transition into a data scientist role.
4. Data engineer
Data engineers work behind the scenes to build data pipelines and systems that collect, aggregate, and transform data into formats other stakeholders can use. Data engineering roles cut across software engineering and database management. Larger organizations have specialized data engineering roles, whereas smaller companies might rely on a software engineer specialized in data to work the data engineer role.
What are data engineers’ responsibilities?
The primary responsibility of a data engineer is to ensure that data is readily available, secure, and accessible to relevant data teams when needed. They may also:
- Build and maintain data pipeline infrastructure for efficient extraction, transformation, and loading (ETL) of data from various sources to data warehouses
- Develop data tools and APIs for other data consumers (data analysts and data scientists) to interact with organizational data
- Ensure data accessibility, quality, and security and implement company data policies concerning data privacy and confidentiality
- Collaborate with engineering teams, data scientists, and other relevant stakeholders to understand how the organization can leverage data to meet its needs
What skills do data engineers need?
To perform their responsibilities efficiently and effectively, data engineers must possess the following technical and soft skills:
- Data wrangling, integration, and warehousing: Data engineers are responsible for collecting, cleansing, transforming, and integrating large volumes of data. You’ll need experience with data migration techniques and data warehousing solutions.
- Databases: Data engineers have a deep understanding of database systems for both SQL and NoSQL databases and are experienced in writing queries to retrieve data.
- Data processing: Data engineers have familiarity with a variety of data processing methods, including batch and real-time processing, and they work with data processing tools like Apache Kafka and Apache Spark.
- Programming languages: Data engineers are typically proficient in at least one programming language, which they use to create algorithms and software solutions to help data teams. Python and Java are popular.
- Basic machine learning understanding: Although not necessary, data engineers with knowledge of data modeling and statistical analysis can help create solutions that are usable by data scientists.
LinkedIn Learning offers several courses if you are looking to improve your data engineering skill set. Our users have also enjoyed the analytics engineering guide from DBT, and all of our technology partners host great insights.
5. Machine learning engineer
Machine learning (ML) engineers work with data scientists to deploy ML models to production environments. In addition, they are responsible for scaling machine learning models for software development purposes.
What are machine learning engineers’ responsibilities?
Machine learning engineers serve as a link between data scientists and software developers. Their responsibilities include:
- Wrapping ML models as APIs and packages that developers integrate into software applications
- Researching and implementing ML algorithms and prototypes from data scientists
- Building ML pipelines that automate ML systems’ training, testing, and deployment
- Identifying differences in data distribution that could impact model performance when deploying it in real-world scenarios
What skills do machine learning engineers need?
Machine learning engineers’ skill sets cut across software engineering and data science. Some of the skills needed to succeed in this role include:
- Programming experience: ML engineers often work with programming languages like Python and Java to turn models into APIs and create ML pipelines.
- ML frameworks: Experience working with frameworks like TensorFlow and Keras.
- Big Data: Knowledge of Big Data frameworks like Hadoop and Spark help ML engineers build pipelines for testing and training data on ML models.
- Communication and collaboration: ML engineers work with data scientists and software developers, so gathering requirements, communicating results, and collaborating are essential.
- Mathematics and statistics: Like data scientists, ML engineers should understand mathematical and statistical methods to help interpret data and solve problems.
Andrew Ng, the founder of Deep Learning AI, offers a machine learning engineering specialization course to help you learn more about the field.
6. Analytics engineer
Analytics engineers clean, transform and document data models to be ready for consumption by the different teams that require it. They sit at the intersection of data engineers and data analysts and use the data pipelines created by data engineers to build useful, well-tested, and documented dataset infrastructure and tools that data analysts and other consumers can use to analyze data.
What are analytics engineers’ responsibilities?
Analytics engineers are responsible for designing and implementing the tools necessary to consume data easily. Some of their responsibilities include:
- Providing clean and transformed data sets ready for analysis
- Maintaining data documentation and definition.
- Developing and maintaining data-related tools and systems
- Working with data analysts and other stakeholders to understand their data needs and ensure that the data infrastructure meets those needs.
What skills do analytics engineers need?
Analytics engineers usually require a mix of data analytics and engineering skills and possess knowledge of how data flows in an organization. Some of the skills needed to succeed in this role include:
- Data querying and SQL skills: Analytics engineers spend a great deal of time exploring data sets and developing logic for transformations, so strong SQL skills are essential for this role.
- Big data stores: Analytics engineers are proficient in various SQL and NoSQL databases, data warehouses, and data lakes and know how to use them.
- Programming language: Analytics engineers typically need strong programming skills in order to design and implement the data infrastructure necessary to transform data for analysis. Analytics engineers may use a variety of programming languages and tools in their work, including Python, and Java, among others.
- Data modeling: Data modeling skills are also useful for analytics engineers as they can use data models to represent data sets in a form that data analysts can easily understand.
- People-skills: Analytics engineers will need to be able to align and communicate with stakeholders from all parts of the organization, with both technical and non technical professionals. So they must be able to resolve conflicts and problem misframings between parties. Great communication and ability to make the complex simple (but no simpler) will be hugely advantageous in this role.
Here are some excellent resources to help you prepare for an analytics engineering role.
- https://analyticsengineers.club
- Analytics engineering boot camp (Paid)
- Analytics engineering with dbt (Paid)
What is the best data job to choose?
The data analyst role is a good fit if you enjoy crunching data to find insights and identify trends and working with various stakeholders to figure out their business questions (the question behind the question). However, if you have a strong quantitative background and like looking for ways to leverage machine learning models to make predictions from data, then the data science role is for you.
If you have a software engineering background and enjoy building data access and ML tools, you should consider the data/ML/analytics engineering role. But a data architect role will be a good fit if you are experienced in the data industry and are interested in leading a data team to define the organization’s overall data strategy.
Remember, the key to succeeding in any data role is to look for ways in which data can solve problems and communicate the insights found to the teams who can act on it. The technical skills needed will vary depending on the company but being skilled in database systems and a programming language provides a solid foundation.
Avo helps teams like Adobe, Rappi, Delivery Hero, and more to plan, implement, and verify their analytics events and workflows faster with better-quality data. If you want to join a space for professionals who design, create, or consume, we’d love for you to join our Slack channel.
Block Quote